IN A NUTSHELL
1. About This Work
Virulence factors (VFs) in Gram-negative bacteria enable pathogens to infect their hosts. A wealth of individual, disease-focused studies have identified a wide variety of VFs, and the growing mass of bacterial genome sequence data provides an opportunity for computational methods aimed at predicting VFs. Despite their attractive advantages and performance improvements, the existing methods have some limitations and drawbacks. Firstly, as the characteristics and mechanisms of VFs are continually evolving with the emergence of antibiotic resistance, it is more and more difficult to identify novel VFs using existing tools that were previously developed based on the outdated data sets; Secondly, few systematic feature engineering efforts have been made to examine the utility of different types of features for model performances, as the majority of tools only focused on extracting very few types of features. By addressing the aforementioned issues, the accuracy of VF predictors can likely be significantly improved. This, in turn, would in particular be useful in the context of genome wide predictions of VFs.
In this work, we comprehensively explore a wide range of various types of heterogeneous features based on an enlarged, up-to-date dataset assembled from several public databases and the recent literature. Specifically, seven popular machine learning algorithms consisting of four classical machine learning algorithms including random forest (RF), support vector machines (SVM), extreme gradient boosting (XGBoost) and multilayer perceptron (MLP), and three deep learning algorithms, including convolutional neural networks (CNN), long short-term memory networks (LSTM) and bi-directional long short-term memory networks (BiLSTM) are employed to train 62 single-method baseline models using these features. Moreover, we effectively combine these baseline models in a deep learning-based hybrid framework (termed DeepVF) using the stacking strategy in order to integrate their individual prediction strengths. The resulting model is shown to be able to accurately predict VFs for Gram-negative bacteria. Extensive experimental results demonstrate the effectiveness of DeepVF: it achieves a much better performance compared to single-method baseline models on the benchmark dataset, and clearly outperforms state-of-the-art VF predictors on the independent test. Using the proposed hybrid ensemble model, a user-friendly online predictor of DeepVF is implemented and can be used as a useful tool for screening and identifying potential VFs in Gram-negative bacteria from sequences.
2. Architecture
The overall workflow of the development of DeepVF can be summarized by the following five steps, which include: (1) data collection and curation; (2) feature extraction; (3) model training and parameterization; (4) integrative model construction, and (5) performance assessment.
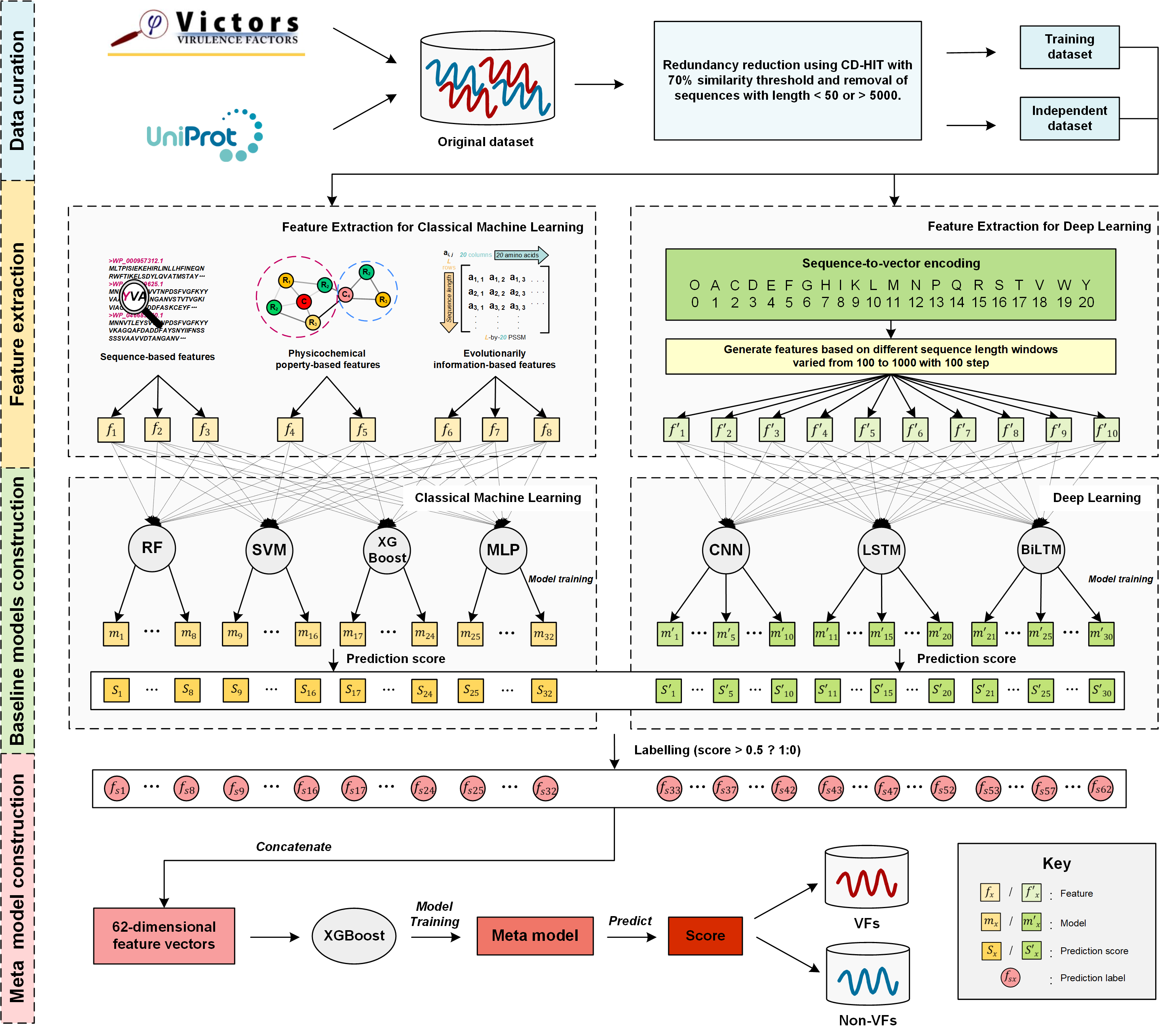
DATASETS
In this work, we constructed an up-to-date dataset for experimentally validated VFs, collected from a recently published database of VFs (Victors) (Sayers, et al., 2019) for multiple bacterial pathogens. 3488 VFs for 36 Gram-negative bacteria pathogens (e.g. acinetobacter baumannii, actinobacillus pleuropneumoniae, escherichia coli and so on) were collected as positive samples. For negative samples, 6845 non-VFs were retrieved from UniProt (UniProt, 2015) using the keyword search (e.g. "not virulent", "not virulence" for Gram-positive bacteria species). Next, the CD-HIT (Huang, et al., 2010) program was applied to cluster the sequences and remove the redundancy in both the positive and negative datasets at the threshold of 70%. In addition, sequences with lengths less than 50 and over 5000 were also removed. The resulting non-redundant dataset contained 1671 VFs and 3661 non-VFs. Subsequently, to construct the final dataset we randomly selected the same number of non-VFs as there are VFs. The ratio of the positive to negative samples was 1:1 so as to avoid a potential bias in the model training. Furthermore, to train the model and validate its generalization ability, 1400 VFs and 1400 non-VFs were randomly selected as the training dataset, while the remaining 271 VFs and 271 non-VFs were used as the independent test dataset.
ONLINE WEB SERVER
1. DeepVF
To maximize user convenience, particularly for the amenability of biomedical scientists and biotechnologists, this user-friendly and publicly accessible web server has been established for the wider research community to perform predictions of novel putative VFs in Gram-positive bacteria. DeepVF is a user-friendly and effective platform hosted by the Monash University cloud computing facility, freely accessible at http://deepvf.erc.monash.edu/.
2. Using the DeepVF web server
As an online server implemented with a user-friendly interface, DeepVF is very easy to use. Users can submit query sequence data in the two following ways: (i) fill in the sequence input form, or (ii) upload a query sequence file in the raw or FASTA format. After the job submission, the web server will provide a unique URL link to users to view the results. The user will also be given the option to fill in the mailbox, via which the user will receive an email containing the URL link for retrieving the prediction output upon the completion of the submitted task.
2.1 Input Formats
Two types of input are accepted by DeepVF: sequences in FASTA format (strongly recommended) and raw sequences.
In the case of input sequences in the FASTA format, you can prepare and input them as follows:
In addition, the following input sequence, which is in the original format downloadable from the UniProt database:
will be formatted (in order to remove those line breaks within the sequence) as follows:
In the case of raw sequences, you can input them as follows:
which will be formated by DeepVF as follows:
2.2 Input sequence limits
- The length of each submitted sequence should be in the range of 50 and 5000.
- Considering that the prediction is a little bit time-consuming (especially in PSSM profile generation), the current maximum number of sequences allowed for each submission by the DeepVF server should be no more than 5000.
3. DeepVF Prediction Result Instructions
For a computationally predicted protein, the results includes the sequence No, the sequence name, the score of the final hybrid meta model, and predict labels(shown in the following figure).
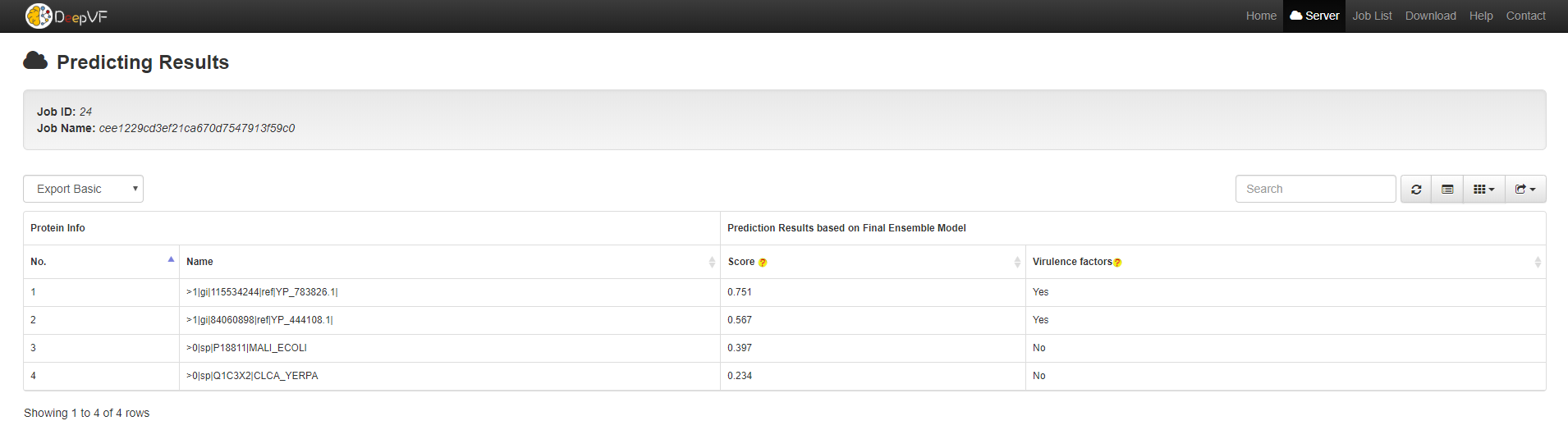